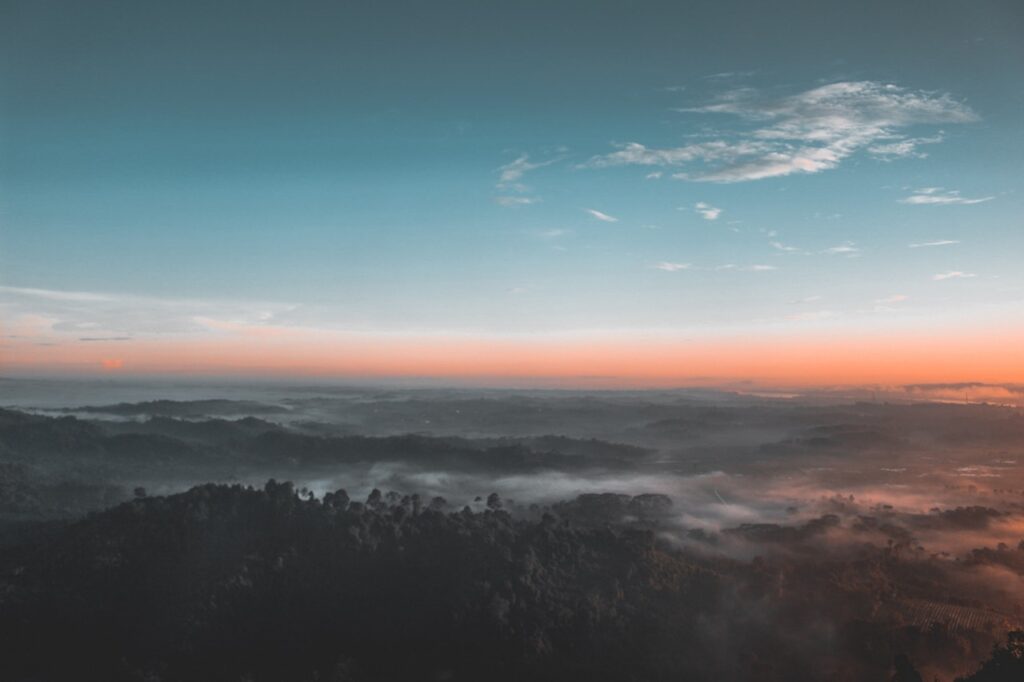
Housing Price Prediction: Part 2
In Part 1 , I explore several important factors that can influence the housing market. For each factor, I dig out the potential relationship between the factor and the housing price index. And understand how these factors impacted the housing price in different historical time frames. If you haven’t get a chance to watch Part 1, please do so, as it will be helpful for understanding Part 2. In this part, I will apply statistical modeling approach to capture the potential relationship between these factors or predictors and Tampa housing price index. And build a predictive model to quantify the relationship. With such model, we can simulate the potential market scenarios in the future, and predict how the housing price would change accordingly!
I use the regression modeling approach here. Through the whole process, I created and selected 10ish predictors through feature engineering and data transformation. These predictors include the ones that can control long term trend, such as population growth index; the predictors that can impact the housing price short term fluctuation, such as unemployment rate, inventory, mortgage rate and some threshold values of these factors; also, there are predictors that can capture seasonality and economic cycles. The model aggregates these predictors with positive and negative weights, and gives final prediction result. For example, the weight for population growth index is positive, has an increasing effect if population grows, unemployment rate has negative weight, has a decreasing effect if unemployment rate is hiking. So, for a future time point, if the increasing effect from population growth is greater than the decreasing effect from unemployment rate increases, then housing price will increase. Vice versa. I use the data from 1995 through 2021 for model development, and 2022 data for model validation. Then I use the model to predict the monthly housing price index for the next two years.
Before final prediction, we need to forecast the future values of each predictor. Here I use time series model to forecast the future values of predictors that demonstrate strong seasonality and cyclic patterns. Three predictors fall into this type: unemployment rate, Hillsborough county housing sales inventory, US new home inventory. Let’s first take a look at the Hillsborough county’ unemployment rate forecast. The blue colored curve represents historical actual values, red colored curve shows the forecast for future. We can tell that the forecast is optimistic. Unemployment rate is projected low. But any forecasts should have a range. Statistically, we call such range as confidence interval. I calculate the 95% confidence interval for the forecast. The two dashed curves on the chart shows the upper bound and lower bound of the forecast range. In layman’s language, the 95% confidence interval means: by the end of 2024, there is 95% chance that the unemployment rate would be within the range of 1% to 5.1%. So the confidence level is 95%. The red colored curve shows the middle value. That’s quite a big range, isn’t it? Some people might ask: is there a narrower range forecast? Of cause yes! But the confidence level will be lower! For example, the 80% confidence interval for the unemployment rate forecast is 1.5% to 3.9%. Forecast without a confidence interval will be fool! So if someone tell you that the crystal ball says housing price will increase or drop at certain time points, increase or drop by x% specifically, but not showing a confidence interval, then it is bullshitting!
Similar to the unemployment rate forecast, we can also forecast Hillsborough sales inventory and US new home inventory. We can see these also have a wide range. But the red colored middle curve kind of represents an optimistic scenario. If we assume: red colored curves’ forecasts would be truly happening, and the Hillsborough county’s population growth rate is 1.5% annually, Hillsborough county median household income increases by 5% every year, mortgage rate would keep increasing but not go beyond 10%, and in Spring 2024 it starts to decrease slowly after breaching 9%. Then the model will give us such forecast: after the housing price decline in Q4 2022, in Q1 2023 it would be more or less flat, and starting Q2 2023 the housing price index would show stable increasing pattern; compared with the summer 2022, end of 2023 price index would slightly increase 2%, summer 2024 would increase 10%. Keep in mind, there is a range. Here is the 95% confidence interval. Based on this confidence interval, we can see it is possible that end of 2023 could even drop 4%, compared to summer 2022. But in general, this is a prediction based on an optimistic scenario. Every coin has two sides. And the chance of another recession coming up is not zero. So, let do an extreme test. Assume a worst case scenario, and see what prediction outcome this model would show. The worst case scenario I define is: the population growth slows down in the future two years, annual growth rate is 0.4%; and a recession like 2008 crisis starts happening in 2023 and end around summer 2024, unemployment rate rapidly increases by around 0.26% monthly, Hillsborough county and the whole country’s housing sales inventory also hikes fast till a level similar to that of 08 crisis, mortgage rate gradually climb to around 10% and slowly decrease in 2024. In this scenario, we can see that there will be a considerably housing price decline trend. Compared to summer 2022, end of 2023 price index drop could be almost 30%, close to the level of Spring 2021; end of 2024 price index could drop to the bottom and reach the level of Q1 2021.
Based upon this model and prediction, we could derive these conclusions: (1) regarding the short term factors like unemployment rate and housing sales inventory, assuming no exogenous factors’ interference, the forecast outcome for 2023 and 2024 looks optimistic, range around the level of 2022; (2) but the forecast range is wide, the 95% confidence interval indicates that unemployment rate and inventory could also increase; (3) in the optimistic scenario, based on 95% confidence interval, compared to summer 2022, Tampa end of 2023 housing price index’s change could range between -4% and 8%, end of 2024 index change could range between 3% and 18%; (4) in the worst case scenario, compared to summer 2022, Tampa end of 2023 index change could range between -27% and -7%; 2024 price index could even drop as much as 30%; (5) to stress again, all models are wrong, but some are useful! (6) and to obtain more reliable predictions, we need to monitor the market and refresh the forecast with updated data on regular basis.